AI-powered Predictive Analytics
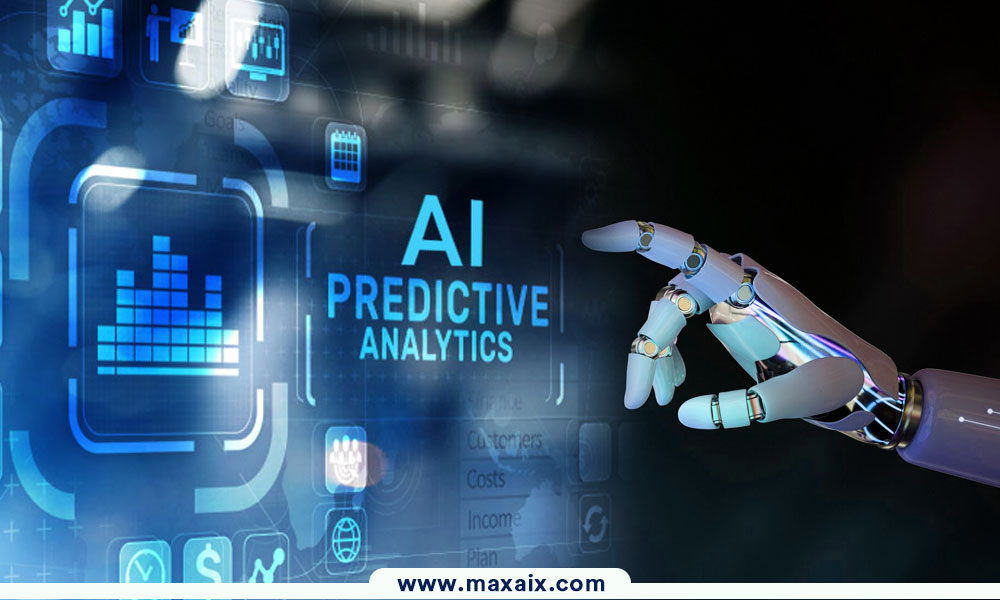
AI-powered Predictive Analytics: Artificial Intelligence (AI) refers to the simulation of human intelligence in machines that can be designed to think and learn like people. These machines can carry out obligations that normally require human intelligence, including visual belief, speech reputation, choice-making, and language translation. AI encompasses an extensive range of technology, including machine learning, natural language processing, robotics, and more.
Evolution of Artificial Intelligence
The concept of Artificial Intelligence dates back to the mid-20th century, with pioneers like Alan Turing and John McCarthy laying the groundwork. Over many years, AI has advanced from easy rule-based totally systems to sophisticated algorithms able to process huge amounts of statistics and make complicated selections. Today, AI is a crucial part of diverse industries, riding innovation and efficiency throughout multiple domain names.
Types of Artificial Intelligence
Narrow AI (Weak AI): This sort of AI is designed to perform a particular mission, which includes facial reputation or language translation. It operates within a restricted scope and can not carry out obligations outdoor its predefined competencies.
General AI (Strong AI): General AI refers to systems that own the capacity to recognize, examine, and follow understanding throughout a wide variety of duties, much like human intelligence. This kind of AI remains at the theoretical level and has now not been realized.
Superintelligent AI: This hypothetical shape of AI surpasses human intelligence in all factors. It is the subject of much debate and speculation, with ability implications for society and ethics.
Importance of AI in Modern Technology
AI is revolutionizing diverse sectors with healthcare, finance, education, and manufacturing. It enables organizations to automate approaches, gain insights from statistics, and enhance selection-making. With advancements in machine learning and deep studying, AI is becoming extra reachable and is playing a critical position in shaping the destiny of the era.
What are Predictive Analytics?
Predictive Analytics involves the use of statistical techniques and algorithms to research historical data and make predictions approximately future occasions. By figuring out patterns and traits in records, predictive analytics enables corporations to forecast results and make knowledgeable selections. It combines records mining, system mastering, and statistical modeling to expect future behavior, risks, and opportunities.
Components of Predictive Analytics
Predictive Analytics is constructed on several key components:
- Data Collection: The manner starts with gathering relevant ancient records from numerous resources. These facts serve as the muse for constructing predictive models.
- Data Preprocessing: Raw information is frequently incomplete, noisy, or inconsistent. Data preprocessing entails cleansing and transforming the statistics to ensure it is suitable for evaluation.
- Model Building: Statistical models or gadget learning algorithms are advanced to discover styles within the records and make predictions.
- Model Validation: Once a model is constructed, it is established against a separate dataset to ensure its accuracy and reliability.
- Deployment: The final version is deployed to make real-time predictions and offer actionable insights.
Techniques Used in Predictive Analytics
Predictive analytics employs various techniques to derive insights from statistics:
- Regression Analysis: Used to apprehend the connection among variables and are expecting non-stop consequences.
- Classification: A technique used to categorize information into predefined classes or classes.
- Clustering: Involves grouping comparable records points together based totally on positive characteristics.
- Time Series Analysis: Analyzes information factors gathered over the years to forecast destiny tendencies.
- Decision Trees: A graphical illustration of choice-making strategies, used to classify and predict outcomes.
The Role of Data in Predictive Analytics
Data is the cornerstone of predictive analytics. High-exceptional, relevant facts are important for building correct models and making dependable predictions. Organizations often leverage large data, combining established and unstructured records from multiple sources to enhance their predictive competencies.
AI for Predictive Analytics
The Intersection of AI and Predictive Analytics
AI-powered predictive analytics are closely intertwined, with AI technologies playing a pivotal function in improving predictive skills. AI for predictive analytics entails the use of machine learning algorithms and artificial intelligence strategies to investigate records, become aware of styles, and make predictions. AI-powered predictive analytics can procedure significant quantities of information, study from it, and constantly improve its accuracy through the years.
How AI Enhances Predictive Analytics
- Automation of Data Analysis: AI algorithms can routinely examine large datasets, eliminating the need for guide records processing.
- Improved Accuracy: AI models can pick out complicated patterns and relationships in information that conventional strategies may pass over, main to extra correct predictions.
- Scalability: AI can deal with enormous quantities of statistics, making it best for businesses dealing with large amounts of information.
- Real-Time Predictions: AI-powered predictive analytics can provide real-time insights, permitting companies to respond fast to changing situations.
AI Techniques in Predictive Analytics
- Machine Learning: Machine learning algorithms, along with choice trees, random forests, and help vector machines, are used to build predictive models.
- Deep Learning: Deep learning of, a subset of machine learning use of neural networks to research information and make complicated predictions.
- Natural Language Processing (NLP): NLP techniques are used to investigate and extract insights from unstructured textual content records.
- Reinforcement Learning: This technique entails education models to make selections primarily based on trial and mistakes, constantly enhancing through the years.
The Benefits of AI in Predictive Analytics
Integrating AI into predictive analytics offers several benefits:
- Enhanced Decision-Making: AI-driven insights permit groups to make extra informed and strategic selections.
- Cost Savings: By automating facts analysis, AI reduces the need to guide hard work, leading to fee savings.
- Increased Efficiency: AI models can analyze records and make predictions faster than conventional techniques, improving overall performance.
- Personalization: AI allows for extra customized predictions, tailoring insights to user needs and choices.
Key Components of AI Predictive Analytics
Data Collection and Management
Data Collection is the first and most critical factor of AI-powered predictive analytics. High-nice information is critical for building accurate models. Organizations collect facts from diverse resources, including internal databases, social media, IoT devices, and third-party providers. Data Management entails organizing, storing, and preserving these records to make sure it’s far without difficulty available for analysis.
Data Preprocessing and Feature Engineering
Before feeding data into AI models, it must be preprocessed to dispose of noise, fill in missing values, and normalize variables. Feature Engineering is the system of selecting, enhancing, and creating new capabilities that improve the performance of predictive models. This step is vital in reworking raw facts into a layout that AI algorithms can efficiently analyze.
Model Selection and Training
The next step in AI-powered predictive analytics is selecting the precise version for the undertaking. Depending on the character of the statistics and the prediction necessities, a special system getting to know algorithms or neural networks can be used. Model Training includes feeding the information into the chosen version and permitting it to learn from the styles within the data.
Model Validation and Testing
Once a version is skilled, it must be verified and tested to ensure its accuracy and reliability. Model Validation includes comparing the version’s predictions against the actual sequences using a separate dataset. Testing helps identify any overfitting or underfitting troubles and best tune the verse the version
Deployment and Monitoring
After validation, the predictive version is deployed in a real-world environment where it can begin making predictions. Continuous Monitoring is essential to ensure the model l maintains its accuracy over the years. Organizations must frequently replace and retrain models as new records come to be had or as situations change.
Interpretability and Explainability
In AI-powered predictive analytics, the models mustn’t be simple and accurate but additionally interpretable. Interpretability refers to the potential to recognize how the version makes its predictions, at the same time as explainability involves offering clear and understandable explanations for the model’s outputs. This is mainly critical in industries like healthcare and finance, wherein choices based totally on AI models may have large effects.
How to Perform Predictive Analytics Using AI?
Step 1: Define the Problem and Objectives
The first step in performing predictive analytics using AI is to clearly outline the trouble you want to remedy and the targets you intend to gain. This may involve predicting customer churn, forecasting income, or figuring out ability dangers. Defining the trouble enables selecting the proper statistics and AI techniques for the challenge.
Step 2: Data Collection and Preparation
Once the problem is defined, the subsequent step is to accumulate applicable facts. This involves accumulating historical information associated with the hassle and ensuring that the facts are correct, entire, and relevant. Data coaching consists of cleansing the records, coping with missing values, and appearing function engineering to create the variables wanted for analysis.
Step 3: Choose the Right AI Model
Selecting the right AI model is crucial for accurate predictions. Depending on the nature of the problem, you may pick from various machine learning algorithms such as linear regression, selection timber, or deep-studying models like neural networks. Each model has its strengths and is perfect for one-of-a-kind sorts of predictive duties.
Step 5: Validate and Test the Model
Once the version is educated, it ought to be proven and examined to ensure its accuracy. This includes evaluating the version’s predictions against actual outcomes with the use of a separate validation dataset. Testing helps identify any capability troubles and ensures the version generalizes properly to new, unseen facts.
Step 6: Deploy the Model
After validation, the AI model is prepared for deployment. To this degree, the model is incorporated into the organization’s present systems, in which it could begin making real-time predictions. Deployment also involves putting in place monitoring systems to track the model’s overall performance and making changes as needed.
Step 7: Monitor and Update the Model
The final step is to continuously reveal the version’s overall performance to ensure it remains accurate through the years. As new information becomes available or because the underlying situations change, the version might also need to be retrained or updated. Regular tracking and upkeep are essential to ensure the model’s lengthy-term fulfillment.
Applications of AI Predictive Analytics
Healthcare
AI-powered predictive analytics is reworking healthcare with the aid of permitting early analysis, personalized remedy plans, and better-affected user consequences. For instance, AI models can predict the chance of sickness outbreaks, identify sufferers prone to continual conditions, and optimize treatment protocols. Predictive analytics is also utilized in sanatorium management to forecast patient admissions and optimize useful resource allocation.
Finance
In the finance enterprise, AI predictive analytics is used to evaluate credit score risk, hit upon fraud, and make funding choices. AI models can analyze historic financial statistics to expect marketplace tendencies, examine the creditworthiness of loan applicants, and become aware of suspicious transactions. This helps financial institutions make greater knowledgeable decisions and decrease risks.
Retail
Retailers use AI predictive analytics to optimize inventory management, personalize advertising and marketing campaigns, and enhance customer retention. By analyzing customer behavior and purchasing patterns, AI models can predict calls for products, become aware of capacity stockouts, and tailor promotions to user clients. This complements the general shopping experience and drives income.
Manufacturing
In manufacturing, AI predictive analytics is used to optimize production tactics, lessen downtime, and enhance product fine. AI models can predict device failures before they occur, schedule maintenance, and optimize supply chain operations. This ends in elevated efficiency, reduced prices, and higher product satisfaction.
Marketing
AI predictive analytics is revolutionizing advertising using permitting personalized campaigns, patron segmentation, and focused advertising. AI models can analyze consumer data to predict destiny behavior, identify high-value clients, and optimize marketing spend. This results in greater effective campaigns and higher conversion rates.
Transportation and Logistics
AI predictive analytics is used in transportation and logistics to optimize routes, reduce delivery times, and enhance fleet control. AI models can predict visitor patterns, optimize transport schedules, and decrease gas intake. This leads to price financial savings and progressed client pride.
Applications of AI Predictive Analytics
Healthcare
AI-powered predictive analytics is reworking healthcare with the aid of permitting early analysis, personalized remedy plans, and better-affected user consequences. For instance, AI models can predict the chance of sickness outbreaks, identify sufferers prone to continual conditions, and optimize treatment protocols. Predictive analytics is also utilized in sanatorium management to forecast patient admissions and optimize useful resource allocation.
Finance
In the finance enterprise, AI predictive analytics is used to evaluate credit score risk, hit upon fraud, and make funding choices. AI models can analyze historic financial statistics to expect marketplace tendencies, examine the creditworthiness of loan applicants, and become aware of suspicious transactions. This helps financial institutions make greater knowledgeable decisions and decrease risks.
Retail
Retailers use AI predictive analytics to optimize inventory management, personalize advertising and marketing campaigns, and enhance customer retention. By analyzing customer behavior and purchasing patterns, AI models can predict calls for products, become aware of capacity stockouts, and tailor promotions to user clients. This complements the general shopping experience and drives income.
Manufacturing
In manufacturing, AI predictive analytics is used to optimize production tactics, lessen downtime, and enhance product fine. AI models can predict device failures before they occur, schedule maintenance, and optimize supply chain operations. This ends in elevated efficiency, reduced prices, and higher product satisfaction.
Marketing
AI predictive analytics is revolutionizing advertising using permitting personalized campaigns, patron segmentation, and focused advertising. AI models can analyze consumer data to predict destiny behavior, identify high-value clients, and optimize marketing spend. This results in greater effective campaigns and higher conversion rates.
Transportation and Logistics
AI predictive analytics is used in transportation and logistics to optimize routes, reduce delivery times, and enhance fleet control. AI models can predict visitor patterns, optimize transport schedules, and decrease gas intake. This leads to price financial savings and progressed client pride.
Benefits and Challenges of Using AI for Predictive Analytics
Benefits of AI Predictive Analytics
- Improved Decision-Making: AI-pushed insights allow organizations to make greater knowledgeable and strategic choices.
- Increased Efficiency: AI models can analyze records and make predictions quicker than conventional techniques, improving usual performance.
- Cost Savings: By automating information evaluation, AI reduces the want for guide labor, leading to value financial savings.
- Real-Time Predictions: AI-powered predictive analytics can offer actual-time insights, allowing companies to respond fast to converting situations.
Challenges of AI Predictive Analytics
- Data Quality: The accuracy of AI models depends on the great of the records used. Poor-best information can lead to misguided predictions.
- Complexity: Developing and deploying AI models can be complicated and require specialized capabilities.
- Cost: Implementing AI predictive analytics can be high-priced, requiring full-size funding in technology and expertise.
Overcoming Challenges
- Data Management: Invest in data management practices to ensure the supply of terrific facts.
- Skill Development: Build or acquire the vital capabilities to broaden, install, and hold AI models.
- Cost-Benefit Analysis: Conduct a radical cost-advantage evaluation to decide the go back on funding in AI predictive analytics.
AI Predictive Analytics Use Cases and Examples in Different Industries
Healthcare
AI predictive analytics is extensively used in healthcare for early disease detection, affected user risk evaluation, and customized remedy plans. For example, AI models can are expecting the chance of a affected user growing a continual circumstance primarily based on their scientific records and life-style elements. This enables healthcare providers to interfere early and offer centered care.
Finance
In finance, AI predictive analytics is used to evaluate credit risk, come across fraud, and make investment selections. For instance, banks use AI models to research transaction statistics and identify probably fraudulent activities. This enables reducing financial losses and protecting clients from fraud.
Retail
Retailers use AI predictive analytics to optimize inventory management, customize advertising and marketing campaigns, and improve purchaser retention. For example, AI models can predict calls for merchandise and assist stores to inventory the proper quantity of stock. This reduces the danger of stockouts and overstocking, leading to value savings and better patron delight.
Manufacturing
In manufacturing, AI predictive analytics is used to optimize production methods, lessen downtime, and enhance product excellent. For example, AI models can expect gadget failures earlier than they occur, permitting producers to schedule protection and avoid pricey disruptions.
Marketing
AI predictive analytics is reworking advertising and marketing with the aid of allowing personalized campaigns, patron segmentation, and centered marketing. For instance, AI models can examine client information to perceive high-value clients and tailor marketing efforts to their alternatives. This leads to higher conversion quotes and expanded consumer loyalty.
Transportation and Logistics
AI predictive analytics is used in transportation and logistics to optimize routes, reduce shipping instances, and enhance fleet control. For instance, AI models can predict site visitors’ styles and endorse the maximum green routes for transport vans. This reduces gasoline consumption and improves delivery times.
How to Get Started with AI Predictive Analytics
Step 1: Assess Your Needs and Objectives
The first step in getting commenced with AI predictive analytics is to evaluate your corporation’s desires and objectives. Determine the particular issues you want to remedy and the desires you need to obtain. This will help you pick out the proper AI strategies and gear to your task.
Step 2: Invest inside the Right Technology
To enforce AI predictive analytics, you’ll want the right generation infrastructure. This includes data garage and processing competencies, AI software, and device getting to know tools. Cloud-primarily based answers may be a fee-powerful option for agencies with limited sources.
Step 3: Build a Skilled Team
Implementing AI predictive analytics requires a group with knowledge in records technology, gadget mastering, and AI improvement. Depending on your enterprise’s size and sources, you could want to lease new expertise or upskill your current workforce.
Step 4: Collect and Prepare Data
Data is the inspiration of AI predictive analytics. You’ll want to gather outstanding, relevant facts from numerous resources and put together it for analysis. This includes cleaning the records, managing missing values, and appearing function engineering.
Step 5: Train, Validate, and Deploy the Model
After selecting the version, the following step is to teach it using your facts. Once educated, the model should be tested to make certain its accuracy and reliability. After validation, the version may be deployed in your organization’s systems, where it may begin making real-time predictions.
Step 6: Monitor and Maintain the Model
Finally, it is important to continuously reveal the model’s performance and make modifications as wished. As new statistics turns into to be had, the model can also want to be retrained or up to date to maintain its accuracy.
Maxaix AI Development Services for Predictive Analytics
Maxaix is a main issuer of AI development services, that specialize in custom answers for predictive analytics. With a group of skilled facts scientists, system studying professionals, and software program builders, Maxaix helps groups harness the electricity of AI to power innovation and acquire their commercial enterprise goals.
Custom AI Development
Maxaix offers custom AI development offerings tailored to the particular wishes of each consumer. Whether you’re trying to enforce predictive analytics in healthcare, finance, retail, or some other industry, Maxaix can layout and broaden a solution that meets your specific necessities.
AI-Powered Predictive Analytics Solutions
Maxaix’s AI-powered predictive analytics solutions allow businesses to make facts-driven choices, optimize operations, and improve consumer delight. By leveraging the modern-day AI technology, Maxaix enables customers advantage precious insights from their facts and live ahead of the competition.
End-to-End Services
Maxaix provides quit-to-end offerings, from data series and preprocessing to version development, deployment, and preservation. This guarantees a continuing and successful implementation of AI predictive analytics on your company.
Industry Expertise
With revel in throughout various industries, Maxaix has the information to expand AI predictive analytics answers for healthcare, finance, retail, manufacturing, and extra. Maxaix’s enterprise-specific knowledge ensures that your solution is customized to the particular demanding situations and opportunities of your area.
The Future of AI Predictive Analytics
Emerging Trends in AI Predictive Analytics
The field of AI predictive analytics is unexpectedly evolving, with numerous rising traits shaping its future:
- Explainable AI: As AI models grow more complex, there’s a growing demand for explainable AI, which offers clean and comprehensible reasons for predictions.
- AI-Driven Automation: The integration of AI with automation technologies is allowing extra green and scalable predictive analytics answers.
- Real-Time Predictive Analytics: Advances in AI and big information technology are making real-time predictive analytics extra on hand, allowing groups to make on-the-spot records-pushed selections.
- AI Ethics and Governance: As AI becomes more normal, there’s an increasing recognition of ethical considerations and governance frameworks to make certain responsible use of AI in predictive analytics.
The Role of AI in Shaping Predictive Analytics
AI is ready to play a imperative function in shaping the future of predictive analytics. As AI technology preserve to develop, predictive models will become extra correct, scalable, and capable of managing more and more complex statistics. This will allow companies to make greater unique predictions, optimize their operations, and benefit a competitive edge.
The Impact of AI on Industries
AI predictive analytics is anticipated to have a profound impact on various industries, from healthcare and finance to retail and manufacturing. As agencies increasingly undertake AI-pushed answers, they may be better ready to navigate challenges, seize opportunities, and pressure innovation of their respective fields.
Preparing for the Future of AI Predictive Analytics
To stay in advance of the curve, corporations ought to put money into AI technologies, build a professional personnel, and foster a tradition of innovation. By embracing the future of AI predictive analytics, groups can liberate new opportunities, enhance selection-making, and acquire lengthy-term achievement.
Conclusion
AI predictive analytics is a effective device that enables agencies to make information-pushed choices, optimize operations, and benefit a competitive facet. By leveraging AI technologies, businesses can analyze sizeable quantities of data, identify patterns, and make accurate predictions about future occasions. While implementing AI predictive analytics comes with demanding situations, the blessings a long way outweigh the prices. With the proper method, era, and know-how, agencies can harness the overall capacity of AI predictive analytics to force innovation and attain their enterprise dreams.
Frequently Asked Questions
What industries advantage from AI predictive analytics?
AI predictive analytics is beneficial across various industries, such as healthcare, finance, retail, production, energy, transportation, and telecommunications. It permits corporations in those sectors to make information-pushed decisions, optimize processes, and enhance client pride.
What are the important thing components of AI predictive analytics?
The key additives of AI predictive analytics encompass statistics series, statistics preprocessing, version selection and training, model validation and trying out, deployment, and monitoring. Each aspect plays a crucial position in building correct and dependable predictive models.
How can I get commenced with AI predictive analytics?
To get commenced with AI predictive analytics, examine your business enterprise’s needs, make investments inside the proper generation, build a skilled group, acquire and put together records, pick out the right AI version, and constantly monitor and preserve the version. Working with a companion like Maxaix also can help make sure a successful implementation.
What are the benefits of the usage of AI for predictive analytics?
The advantages of the usage of AI for predictive analytics consist of progressed choice-making, improved efficiency, value savings, personalization, and actual-time predictions. AI permits groups to investigate statistics extra efficaciously and make more knowledgeable strategic choices.
What demanding situations are related to AI predictive analytics?
Challenges related to AI predictive analytics consist of data fine issues, version complexity, interpretability, ethical concerns, and the value of implementation. Overcoming these demanding situations requires cautious making plans, investment in the right technology, and a focus on transparency and ethics.
How does Maxaix guide AI predictive analytics?
Maxaix helps AI predictive analytics by presenting custom AI improvement offerings, AI-powered predictive analytics answers, stop-to-quit offerings, and enterprise information. Maxaix enables corporations harness the power of AI to drive innovation and acquire their enterprise desires.